Hypothesis Testing
Hypothesis Testing
Skills you’ll gain
This course is part of our Lean Six Sigma Green Belt program, which consists of four courses designed to prepare you for the International Association of Six Sigma Certification (IASSC) Green Belt exam. We recommend you take all four courses in the program to be fully prepared for the exam.
Hypothesis testing allows you to make decisions about problems based upon statistically significant data. Depending on the nature of the hypothesis and data available, different tests should be applied.
In this course, you will learn about 20 different statistical tests. You will understand when to use each test, and when not to use them. You will also identify the level of risk associated with different statistics, and how best to work with them.
The training features plenty of opportunities to practice with examples, exercises, and quizzes to test your knowledge. By the end of the course, you will have learned how to apply these hypothesis tests in your business processes.
The course is designed from the standpoint of making sound business decisions, not deriving proofs behind the formulas or statistics. You won't need to do any advanced math, as popular programs like Excel and Minitab will do that for you. While the computer crunches the numbers, you will learn how to read and interpret the test results to understand the messages in your data.
Highlights:
- 34 practical tutorials with videos, reference guides, exercises, and quizzes.
- Designed to prepare you in part for the IASSC Green Belt exam. To prepare in full, you should also take the Lean Six Sigma Principles, Statistical Process Control, and Measurement Systems Analysis courses part of our four-course Lean Six Sigma Green Belt program.
- Understand the concepts of hypotheses in problem-solving.
- Identify the hypothesis testing process, and best practices that should be applied.
- Learn key decision factors for selecting which hypothesis test to use.
- Apply statistical analysis principles such as inferential statistics.
- Learn how to read residual graphs and conduct regression analysis.
- Conduct statistical hypothesis tests including T Tests, F Tests, ANOVA, and more.
- Aligned to the IASSC Lean Six Sigma Green Belt Body of Knowledge.
- The only method to earn an IASSC certification is to successfully sit for and pass an official IASSC certification™ exam, which can be taken through IASSC. We do not provide access to IASSC Certification exams.
- Earn 3 PDUs or contact hours toward your Project Management education for certification with PMI.
Once enrolled, our friendly support team and tutors are here to help with any course-related inquiries.
Syllabus
Download syllabus-
1
Inferential Statistics Hypothesis testing relies on the principle of inferential statistics. A sample data set from a larger population of data is statistically analyzed. The result of the analysis of the sample data is used to infer a conclusion about the larger population of data. This lesson will discuss the concept and ways to compare the data sample and the data population. 6m
-
2
Confidence Intervals Since it is often impossible to analyze all the data items in a population of data, a sample is selected from the data population. But there is a chance that the sample may not perfectly represent the full population. Based upon an understanding of the data sample and population, a range or interval can be established around any sample statistic that represents the boundaries within which the population statistic exists. In this lesson, we learn how to determine the size of that range or confidence interval. 6m
-
3
Samples and Sample Selection Hypothesis testing relies on the use of data samples. However, the power and value of the hypothesis test are based on the size of the sample and the means by which it was selected. In this lesson, we consider factors for selecting sample data points and we determine the size of the sample needed based on the desired accuracy of the answer. 5m
-
4
Standard Error One of the most important statistical measures of a data set is the mean or average value. For inferential statistics to be valid, the mean of the sample should be approximately the same as the mean of the entire population of data. The Standard Error is the measure of how accurately the sample mean will approximate the population mean. In this lesson, we will determine how to calculate the standard error and how the sampling process can affect that error. 5m
-
5
Alpha and Beta Risk The statistic created in a hypothesis test is only 100% accurate for the data in the sample from which the statistic was calculated. The application of the statistical value to the broader data population has some uncertainty. It is possible that the full population of data is different from the sample of data that was tested. This uncertainty gives rise to the Alpha and Beta risks discussed in this lesson. 5m
-
6
Significance and Power The ability of a hypothesis test to provide insight into the characteristics of a data population is based on the sample of data selected and some statistical characteristics of the sample and the population. The relationship between these gives rise to two measures that can be made concerning the validity of the hypothesis test. These measures are Significance and Power and will be discussed in this lesson. 5m
-
7
The P Value Inferential statistics relies on a statistical measure of goodness known as the "P" Value to determine whether to accept or reject the Null hypothesis. This P value is based upon the type of test conducted and the confidence interval and Alpha risk that are applied to the situation. This lesson explains the principle of the "P" value and its use in Lean Six Sigma projects. 5m
-
1
Normal Distribution One of the most important criteria for selecting a hypothesis test is based upon whether the data being analyzed is normal on not normal. The normality question does not prove or disprove the hypothesis, rather it determines the type of statistical test that should be performed. This lesson reviews the concept of normality and how to determine it. 6m
-
2
Non-normal Distribution Non-normal data often has a pattern. Knowing that pattern can help to transform the data to normal data and can aid in the selection of an appropriate hypothesis test. 7m
-
3
Z Transformation One of the techniques used to measure the normality or non-normality is the Z score. This Z score is often used when comparing data sets and in some of the hypothesis test calculations. 5m
-
4
Distributions and Discrete Data Data is often displayed visually in distributions. Recognizing the type of display and the nature of the distribution can aid in the selection and analysis of a hypothesis test. This lesson will explain data distributions and review the typical distributions for discrete data. 6m
-
5
Continuous Data Distributions There are many types of continuous data distributions. These are often associated with physical characteristics of the data or system being studied. The ability to recognize the type of distribution aid in the selection and analysis of a hypothesis test. 4m
-
1
Correlation A common investigation in Lean Six Sigma problem solving is to determine if two factors are correlated. This insight will often point to an underlying cause of the problem. This lesson explains how to do correlation analysis using both Excel and Minitab. It also includes a discussion of the Pearson correlation coefficient. 6m
-
2
Regression Models Regression models are formulas that allow us to predict the performance of the system being analyzed. As a hypothesis test, we can determine whether the regression formula is able to predict the performance of the sample data set. This lesson defines the different types of regression analyses that will be discussed in later lessons and how to choose between the regression approaches. 4m
-
3
Simple Linear Regression Simple linear regression analysis creates an equation that correlates two factors. This equation assists in understanding problems, and it can also be used to manage the problem or process going forward. This lesson shows how to calculate this line with the help of either Excel or Minitab. 6m
-
4
Residual Analysis A statistical analysis or test creates a mathematical model to fit the data in the sample. The real-world data seldom precisely fits the model. The differences between the model and the actual data are known as residuals. This lesson explains how to read residual graphs and analyses. 6m
-
5
Prediction Interval A regression formula can be used to predict how a system responds to various inputs. However, based on the nature of the data set, there will be some uncertainty to the accuracy of that prediction. This lesson shows how to determine that level of uncertainty in the regression model prediction. 4m
-
6
Multiple Linear Regression Many times there are multiple factors that are influencing the response variable in a problem. Multiple regression determines the relationship between the response factor and multiple control factors. Like with simple linear regression, a formula is created that allows both analysis and prediction of the process and problem. 5m
-
7
Non-linear Regression Many of the problems encountered in Lean Six Sigma projects do not have the straight-line correlation effect that we discussed with simple linear regression or multiple regression analysis. The relationship is better modeled by an exponential curve, a parabola, or other non-linear relationship. This lesson will use Minitab to assist in determining the best model to predict performance. 6m
-
8
Box-Cox and Transformations Data transformation can convert a Lean Six Sigma problem from a non-linear regression analysis into a linear regression which is often easier to understand and explain to the stakeholders. The most common transformation approach is the Box-Cox transformation. In this lesson, we demonstrate how this transformation works and discuss when to use it. 4m
-
1
Test of Proportions The One-Sample and Two-Sample Test of Proportions are used with discrete data. These tests determine whether the percentage of a particular attribute being studied is similar to or different from the selected target value. These tests are illustrated using both Excel and Minitab. 7m
-
2
Chi Square When the data is discrete data, but there are more than two samples to be compared, the Chi Square test is used. This test is quickly accomplished using Minitab. It can be done using Excel, but requires several intermediate steps. This lesson explains this test approach. 7m
-
3
One Sample Tests One sample tests are tests of a single dataset that compares the descriptive statistics of that data set against target values. 6m
-
4
Variance Tests Many of the hypothesis test approaches will change depending on whether the continuous data has equal variances or unequal variances between data sets. Therefore, the F Test or Bartlett's Test must be completed to determine if variances are equal. 6m
-
5
T-Tests T-Tests compare the mean of two data samples to each other. 6m
-
6
ANOVA Approach The Analysis of Variance (ANOVA) test is a commonly used test in Lean Six Sigma projects. It allows the comparison of multiple data sets to determine whether there is a statistical difference in those data sets. The analysis can be easily done in both Excel and Minitab. This lesson addresses the basics of ANOVA. 5m
-
7
ANOVA Analysis The ANOVA analysis can be done either numerically with a P value or graphically. The P value will indicate whether at least one of the dataset's means is statistically different. The graphical analysis will show which dataset(s) is different. 7m
-
8
One-Sample Sign The One-Sample Sign Test and the One-Sample Wilcoxon Test accomplish the same purpose, but each has strengths and weaknesses. When the data is not normal, or it is not known that it definitely is normal, these tests can be used to determine if the data set statistics meets or exceeds a target value. The application of this test using Minitab is illustrated in this lesson. 6m
-
9
Levene's and Mann Whitney Tests These tests are used when comparing two non-normal data samples. The Levene's Test checks for the variance and the Mann-Whitney checks the median. These tests are both standard tests in Minitab and the lesson will illustrate how to conduct them. 4m
-
10
Mood’s Median, Kruskal-Wallis, Friedman Tests These three tests are for multiple samples of non-normal data. Each test has its strengths and weaknesses. The appropriate test will depend upon what is known, or not known, about the data in the samples. The Minitab interface to accomplish each of these tests is similar. This lesson will explain the differences and show how to conduct the test and read the results. 7m
Certificate
Certificate of Completion
Awarded upon successful completion of the course.
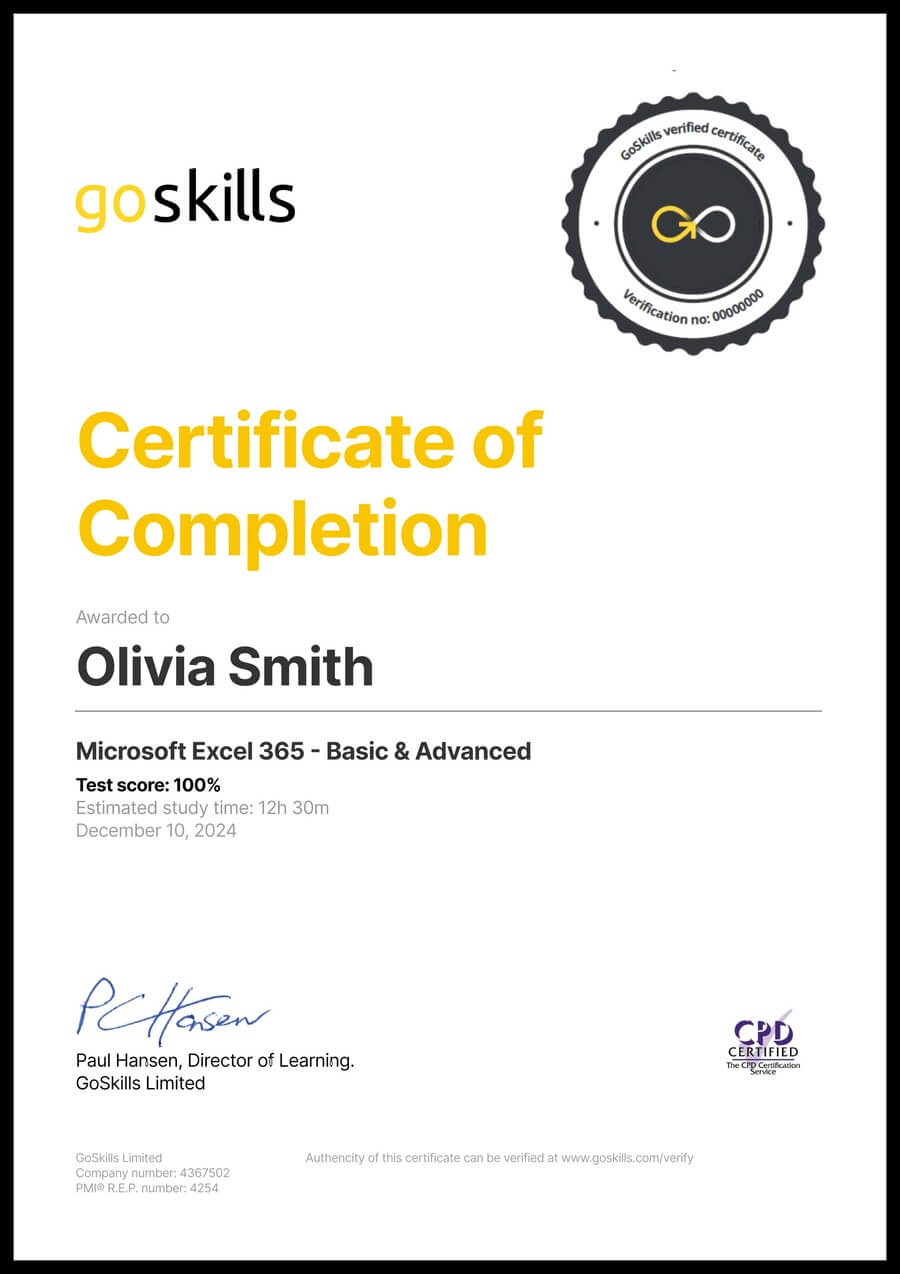
Instructor
Ray Sheen
Ray is a certified Project Management Professional (PMP) with the Project Management Institute, a certified Scrum Master with Scrum Alliance, and certified Lean Six Sigma Black Belt with IASSC. He is a member of the Project Management Institute and the Product Development Management Association.
He is president and founder of Product & Process Innovation, Inc. and is a veteran business leader with over 25 years of executive, project management, and engineering management experience. Ray has worked in several industries including aerospace, electrical distribution and utilities, biotechnology, appliances, electronics, machining, medical devices, pharmaceutical, automotive, and financial services. He has held executive management positions in a Fortune 500 company and has been involved in entrepreneurial startup organizations.
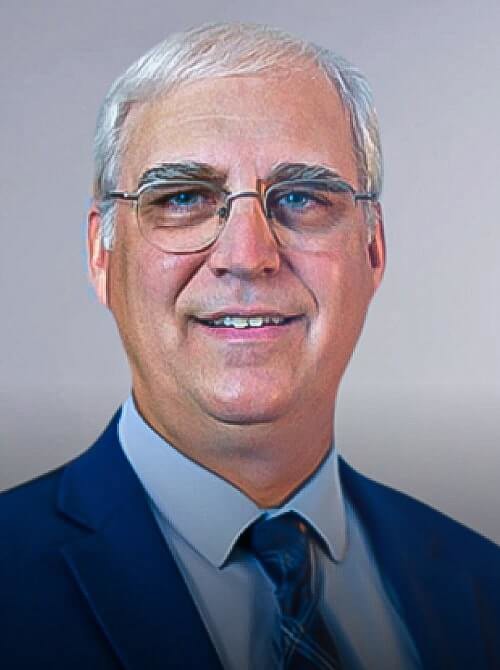
Ray Sheen
Project Management Instructor & Author
Accreditations
Link to awards GoSkills Ltd is an IASSC Accredited Training Organization™. The PMI ATP seal, PMI, PMP, CAPM and PMBOK are registered marks of the Project Management Institute, Inc.How GoSkills helped Chris
I got the promotion largely because of the skills I could develop, thanks to the GoSkills courses I took. I set aside at least 30 minutes daily to invest in myself and my professional growth. Seeing how much this has helped me become a more efficient employee is a big motivation.
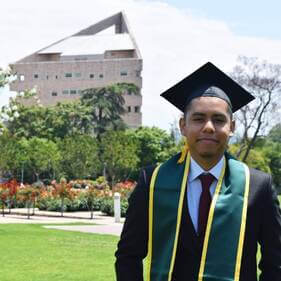